New method for improving nanoparticle size estimation
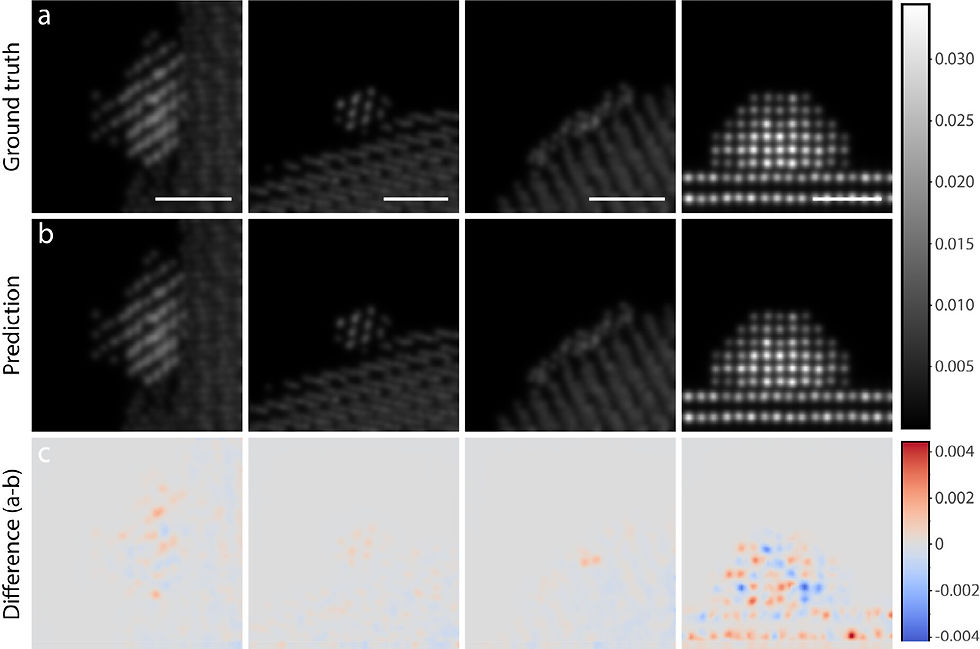
A new method to improve the size estimation of nanoparticles from scanning transmission electron micrographs has been developed by researchers at the Swiss Federal Laboratories for Materials Science and Technology, and at ETH Zürich. The researchers employ a multislice surrogate model to calculate synthetic scanning transmission electron microscopy (STEM) images to train machine learning models for the size estimation of nanoparticles. For small particles, the researchers found that their method produces significantly more accurate nanoparticle size estimation.
They were motivated to explore a new way to find novel atomic-scale characterization techniques for heterogeneous catalysis that can produce more accurate data and can be automated. HAADF-STEM (Hybrid angle annular dark-field scanning transmission electron microscopy) is a powerful tool for imaging surface-supported catalyst species at atomic resolution, where current analysis workflows are either imprecise, specific to single atom catalyst species, or not amenable to experiment automation due to requirements on the orientation of the particle.
They created a surrogate model of multislice image simulations and then trained this model on a set of paired atomic models and multislice HAADF-STEM images of ceria-supported Pt nanoparticles. The surrogate model is a 3D U-Net(conn.) The surrogate model was employed to simulate a big data set of 100k synthetic multislice images of Pt particles on ceria. Next, they used this data set to train a size-estimator network to predict the size of an imaged nanoparticle in number of atoms.
They found that their method particularly increases the accuracy of nanoparticle size estimation for small particles. They found, too, that the generation of a large synthetic data set is more resource-efficient than generating additional real multislice images.
The researchers expect their method will be extremely useful for statistical STEM studies of heterogeneous catalysts to identify structure-property relationships. They expect future improvements of this methodology to come from using a single pixel size (fixed magnification), automatic data acquisition, less noisy experimental data, and constructing more realistic structural models. Future studies could determine what number of multislice images give a sufficiently accurate training of a surrogate model and whether faster alternatives to the multislice algorithm lead to comparable prediction quality and generalization to experimental data. Reference Improving Nanoparticle Size Estimation from Scanning Transmission Electron Micrographs with a Multislice Surrogate Model
Henrik Eliasson, Rolf Erni https://pubs.acs.org/doi/10.1021/acs.nanolett.4c06025 Nanotechnology World (NW)
Comments