Machine learning predicts mechanical properties of porous materials
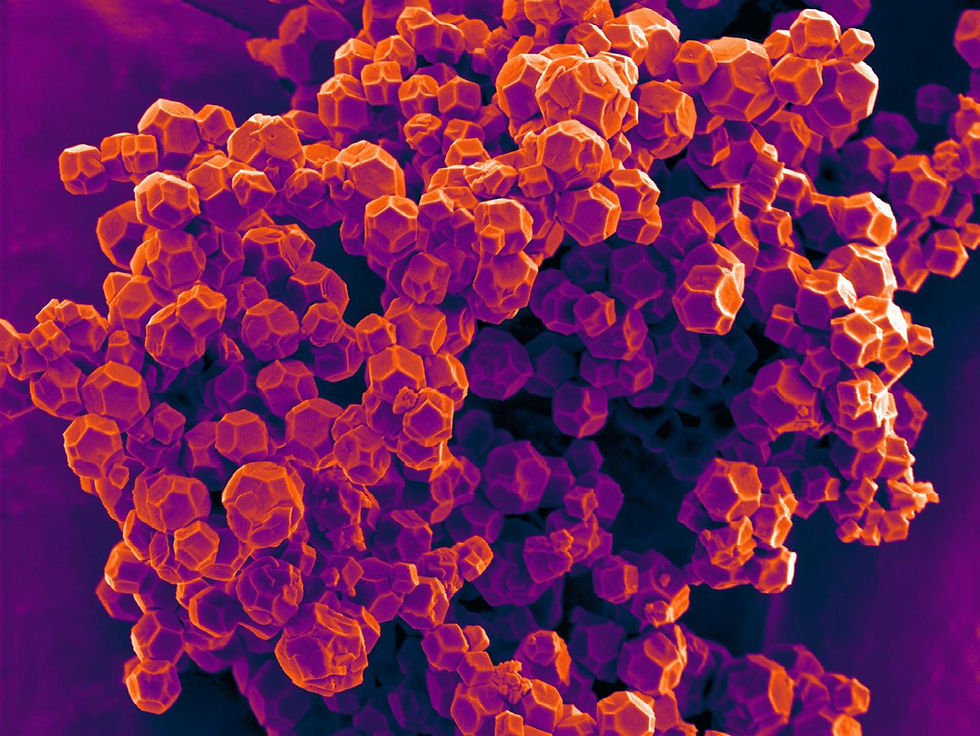
Machine learning can be used to predict the properties of a group of materials which, according to some, could be as important to the 21st century as plastics were to the 20th.
Researchers have used machine learning techniques to accurately predict the mechanical properties of metal organic frameworks (MOFs), which could be used to extract water from the air in the desert, store dangerous gases or power hydrogen-based cars.
The researchers, led by the University of Cambridge, used their machine learning algorithm to predict the properties of more than 3000 existing MOFs, as well as MOFs which are yet to be synthesised in the laboratory.
The results, published in the inaugural edition of the Cell Press journal Matter, could be used to significantly speed up the way materials are characterised and designed at the molecular scale.
MOFs are self-assembling 3D compounds made of metallic and organic atoms connected together. Like plastics, they are highly versatile, and can be customised into millions of different combinations. Unlike plastics, which are based on long chains of polymers that grow in only one direction, MOFs have orderly crystalline structures that grow in all directions.
This crystalline structure means that MOFs can be made like building blocks: individual atoms or molecules can be switched in or out of the structure, a level of precision that is impossible to achieve with plastics.
The structures are highly porous with massive surface area: a MOF the size of a sugar cube laid flat would cover an area the size of six football fields. Perhaps somewhat counterintuitively however, MOFs make highly effective storage devices. The pores in any given MOF can be customised to form a perfectly-shaped storage pocket for different molecules, just by changing the building blocks.
"That MOFs are so porous makes them highly adaptable for all kinds of different applications, but at the same time their porous nature makes them highly fragile," said Dr David Fairen-Jimenez from Cambridge's Department of Chemical Engineering and Biotechnology, who led the research.
MOFs are synthesised in powder form, but in order to be of any practical use, the powder is put under pressure and formed into larger, shaped pellets. Due to their porosity, many MOFs are crushed in this process, wasting both time and money.
To address this problem, Fairen-Jimenez and his collaborators from Belgium and the US developed a machine learning algorithm to predict the mechanical properties of thousands of MOFs, so that only those with the necessary mechanical stability are manufactured.
The researchers used a multi-level computational approach in order to build an interactive map of the structural and mechanical landscape of MOFs. First, they used high-throughput molecular simulations for 3,385 MOFs. Secondly, they developed a freely-available machine learning algorithm to automatically predict the mechanical properties of existing and yet-to-be-synthesised MOFs.
"We are now able to explain the landscape for all the materials at the same time," said Fairen-Jimenez. "This way, we can predict what the best material would be for a given task."
The researchers have launched an interactive website where scientists can design and predict the performance of their own MOFs. Fairen-Jimenez says that the tool will help to close the gap between experimentalists and computationalists working in this area. "It allows researchers to access the tools they need in order to work with these materials: it simplifies the questions they need to ask," he said.
Structure-Mechanical Stability Relations of Metal-Organic Frameworks via Machine Learning Peyman Z. Moghadam, Sven M. J. Rogge, Aurelia Li, Chun-Man Chow, Jelle Wieme, Noushin Moharrami, Marta Aragones-Anglada, Gareth Conduit, Diego A. Gomez-Gualdron, Veronique Van Speybroeck, David Fairen-Jimenez Matter 15 May 2019 DOI: https://doi.org/10.1016/j.matt.2019.03.002
Contact information:
David Fairen-Jimenez
Cambridge's Department of Chemical Engineering and Biotechnology
Tel: 0044 1223 334785
University of Cambridge